Components of Hadoop - An Overview
Blog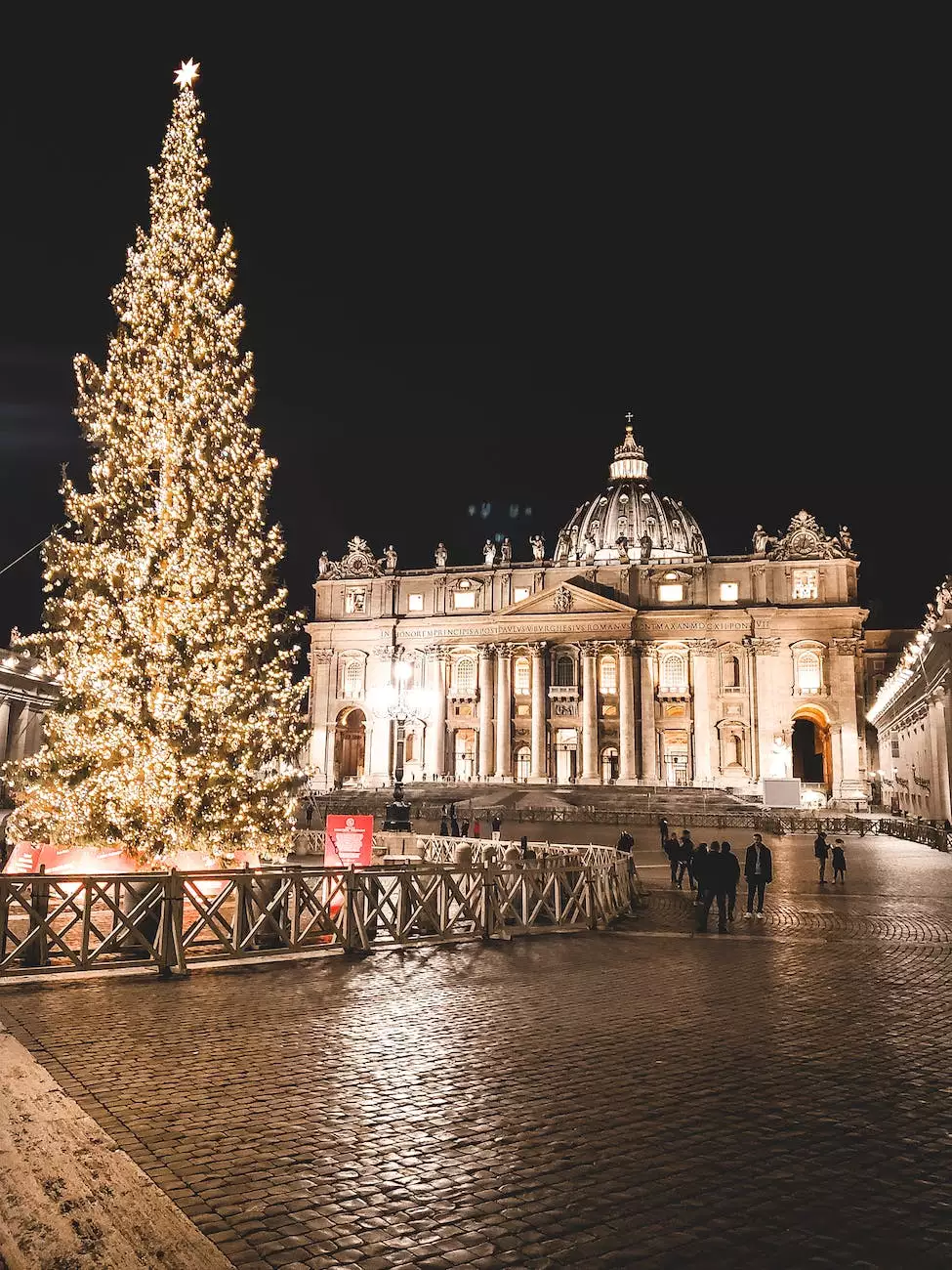
Introduction to Hadoop
Hadoop is a powerful and popular framework used for big data processing and analytics. Developed by the Apache Software Foundation, Hadoop enables the distributed processing of large datasets across clusters of computers. It has emerged as a game-changer in the field of data management, providing scalable and reliable solutions for businesses.
The Core Components of Hadoop
Hadoop Distributed File System (HDFS)
HDFS is a distributed file system that enables Hadoop to store and manage large datasets across multiple machines. It breaks down files into smaller blocks and distributes them across the cluster, ensuring high availability and fault tolerance. HDFS is designed to handle massive amounts of data and provides efficient data storage and retrieval capabilities.
MapReduce
MapReduce is a programming model and algorithm used by Hadoop to process and analyze large amounts of structured and unstructured data. It follows a two-step approach: the map phase and the reduce phase. In the map phase, data is divided and processed in parallel, while in the reduce phase, the results are combined and aggregated. MapReduce enables distributed processing and allows Hadoop to handle complex data processing tasks efficiently.
YARN (Yet Another Resource Negotiator)
YARN is a resource management framework in Hadoop that enables multiple applications to run and share resources on the same cluster. It separates the resource management and job scheduling functionalities, providing a flexible and scalable platform for running various workloads. YARN plays a crucial role in optimizing resource utilization and improving the overall performance of Hadoop.
Hadoop Common
Hadoop Common provides the essential libraries and utilities required by other Hadoop modules. It includes the necessary Java libraries and files that serve as the foundation for Hadoop's functionality. Hadoop Common ensures compatibility and consistency across different Hadoop components, making it easier for developers to build Hadoop-based applications.
The Ecosystem Components of Hadoop
Hive
Hive is a data warehousing and SQL-like query language for Hadoop. It provides a high-level interface that allows users to write SQL queries to analyze and process large datasets stored in Hadoop. Hive translates SQL queries into MapReduce jobs, making it easier for non-programmers to interact with Hadoop and perform data analysis tasks.
Pig
Pig is a high-level scripting language designed for data analysis and manipulation in Hadoop. It provides a set of operators and functions that simplify complex data transformations. Pig Latin, the language of Pig, allows users to express data operations concisely, abstracting the underlying implementation details. Pig is widely used in the Hadoop ecosystem for ad-hoc data processing and ETL (extract, transform, load) operations.
HBase
HBase is a distributed, scalable, and consistent NoSQL database that runs on top of Hadoop. It provides random read and write access to large datasets, making it suitable for real-time applications that require low-latency data retrieval. HBase is commonly used for storing and managing structured data in Hadoop, especially in scenarios where random access to large datasets is critical.
Spark
Spark is a lightning-fast cluster computing system that complements Hadoop's batch processing capabilities. It is designed for in-memory data processing and enables real-time stream processing, interactive queries, and iterative machine learning. Spark integrates seamlessly with Hadoop and provides APIs for programming in Java, Scala, Python, and R. It has gained popularity due to its speed, ease of use, and support for advanced analytics.
ZooKeeper
ZooKeeper is a widely-used coordination service for distributed systems. It provides a centralized infrastructure that helps in maintaining configuration information, naming, synchronization, and group services. ZooKeeper ensures the availability, reliability, and consistency of Hadoop clusters by coordinating and managing various distributed processes.
Impala
Impala is an open-source, massively parallel processing (MPP) query engine for Hadoop. It provides interactive, low-latency SQL queries directly on Hadoop, eliminating the need for data movement or separate extract-transform-load (ETL) steps. Impala allows users to query data stored in HDFS, HBase, and other Hadoop-supported file formats, making it ideal for real-time analytics and exploration.
Conclusion
The components mentioned above collectively form the powerful Hadoop ecosystem, enabling businesses to process, analyze, and gain valuable insights from massive datasets. Understanding the role and functionality of each component is crucial in leveraging the full potential of Hadoop for your business needs. By harnessing the capabilities of Hadoop and its ecosystem, businesses can make informed decisions, optimize processes, and unlock new avenues of growth in the digital era.
Your SEO Geek - Leading SEO Agency in Buffalo, NY. We are the experts you can rely on for all your digital marketing needs. Contact us today to boost your online presence and drive more organic traffic to your website.