Harnessing the Power of Spam Filtering Machine Learning
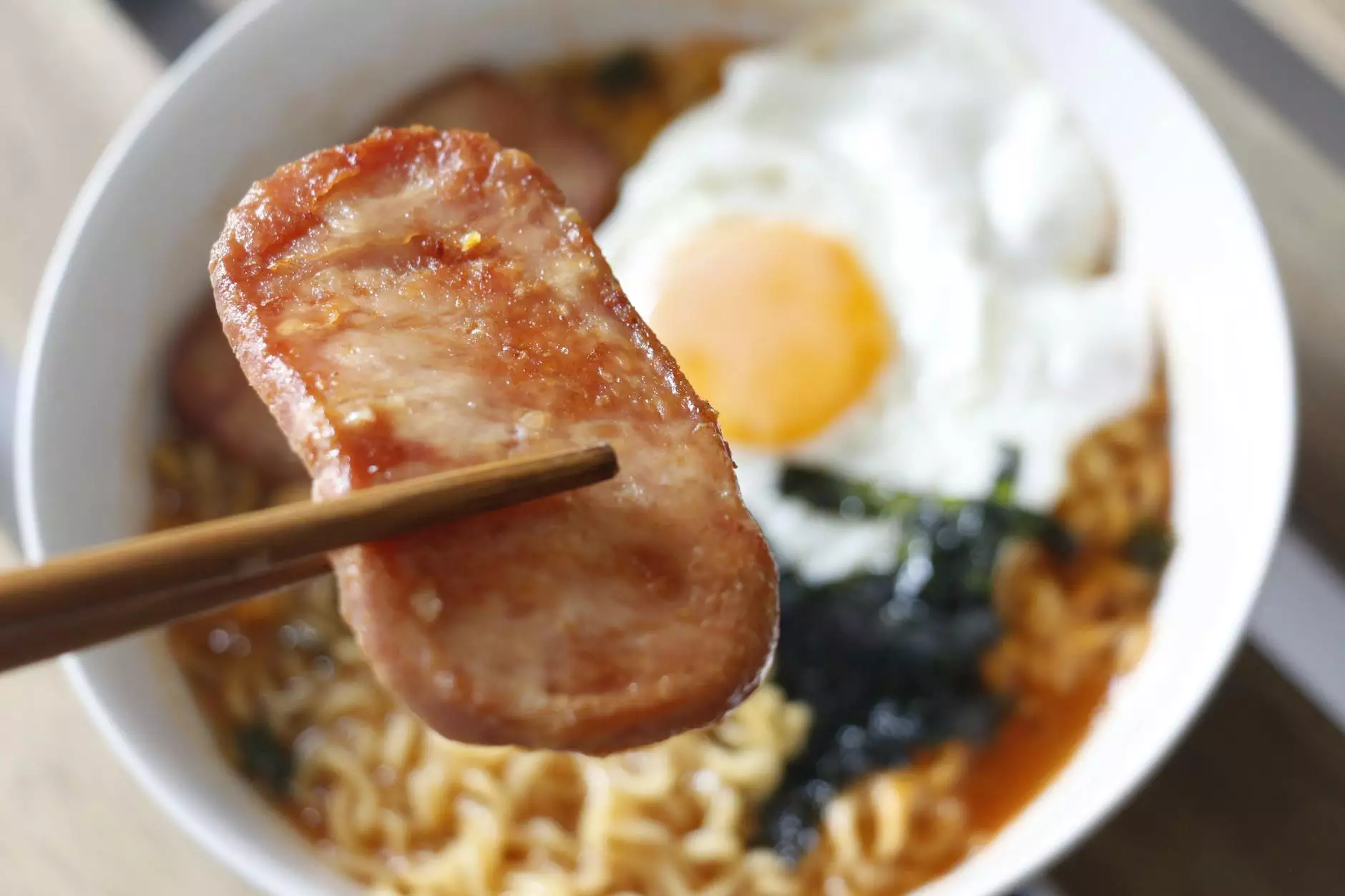
In the modern digital landscape, businesses are continuously striving to enhance their operational capacities and fortify their data security measures. One of the most pressing issues in this domain is spam filtering. With the advent of machine learning, this complex challenge has found innovative solutions that not only save time but also significantly improve email security protocols. In this article, we will delve into the intricacies of spam filtering machine learning, exploring its applications, benefits, and implications for IT services and security systems.
Understanding Spam Filtering
Spam refers to unsolicited, irrelevant, or inappropriate messages sent over the internet, typically to a large number of users. These messages can clutter inboxes, diminish productivity, and pose significant security risks, including phishing attacks and malware distribution. Effective spam filtering is critical for any business's IT infrastructure.
Traditional vs. Modern Spam Filtering Approaches
Historically, spam filters operated based on predefined rules and keyword matching. While effective to some extent, this method often resulted in high false positives — legitimate emails incorrectly marked as spam. The evolution of machine learning has revolutionized spam filtering by introducing algorithms that can learn from data patterns and improve over time.
- Rule-based Filtering: Uses static rules to identify spam, such as specific words or phrases.
- Blacklisting: Blocks known spam sources by maintaining lists of domain names and IP addresses.
- Machine Learning: Employs algorithms that adapt and learn from new data to distinguish between spam and legitimate communication.
The Role of Machine Learning in Spam Filtering
Machine learning algorithms analyze vast datasets to identify characteristics of spam emails, enabling a more accurate filtering process. With the capability to adapt to new tactics employed by spammers, these systems continuously improve their detection rates.
How Machine Learning Algorithms Work in Spam Filtering
The process typically involves the following steps:
- Data Collection: Gathering a comprehensive dataset that includes both spam and legitimate emails for training.
- Feature Extraction: Identifying unique features and patterns that differentiate spam from non-spam. Features may include the frequency of certain words, email metadata, and user behavior.
- Training the Model: Using labeled datasets to train the model on how to classify emails effectively. Various algorithms can be applied, including Naive Bayes, Support Vector Machines, and Neural Networks.
- Evaluation: Testing the model with a separate dataset to measure accuracy and identify any biases or weaknesses.
- Deployment: Implementing the model into an organization’s email system, where it can start classifying incoming emails in real-time.
- Continuous Learning: The model continuously learns from new data, adapting its predictions based on incoming emails and feedback from users.
Benefits of Machine Learning in Spam Filtering
The incorporation of machine learning techniques into spam filtering systems offers myriad benefits that are essential in today’s cyber environment:
1. Enhanced Accuracy
Machine learning algorithms have the ability to improve accuracy through ongoing training and data analysis. This results in a significant reduction in both false positives and false negatives, ensuring that valid emails reach the inbox while spam is effectively filtered out.
2. Adaptive Learning
As spammers continually evolve their tactics, traditional filters may become ineffective over time. Machine learning enables filters to stay ahead of these changes by learning from new spam tactics, thereby ensuring robust protection against emerging threats.
3. Improved User Experience
With fewer legitimate emails being marked as spam, employees can concentrate on productive tasks without the frustration of sifting through their junk folders. This leads to increased productivity and efficiency within the organization.
4. Efficient Resource Management
A well-implemented spam filtering system reduces the need for extensive manual intervention in email management. Consequently, IT teams can allocate their resources towards other critical areas within the organization.
Implementing Machine Learning for Spam Filtering
To successfully implement a spam filtering machine learning system, businesses must adhere to several best practices:
1. Choose the Right Algorithm
Different machine learning algorithms yield varying results based on the specific datasets and contexts. Organizations should experiment with multiple algorithms to identify the most effective solution for their unique spam filtering needs.
2. Invest in Quality Data
High-quality, well-labeled datasets are paramount for training machine learning models. Businesses should invest time in curating comprehensive datasets to ensure the effectiveness of their spam filtering systems.
3. Regularly Update Models
Spam tactics are constantly evolving. Organizations must regularly update their machine learning models with new data and retrain them to maintain accuracy and relevance.
4. Monitor Performance Indicators
Establishing clear performance metrics is essential for measuring the success of the spam filtering system. Metrics such as detection rate, false positive rate, and user feedback should be continuously monitored and analyzed.
Case Studies: Effective Spam Filtering Machine Learning Implementations
Numerous businesses have experienced significant improvements in their email security through the implementation of spam filtering machine learning. Below, we highlight a few case studies.
Case Study 1: TechCorp Solutions
TechCorp Solutions, a mid-sized IT service provider, faced a substantial influx of spam, leading to decreased employee productivity and increased cybersecurity risks. By employing a machine learning algorithm to filter emails, TechCorp reduced spam infiltration by over 90%. The system also provided weekly reports, enabling the IT team to understand emerging spam trends and adjust their strategies accordingly.
Case Study 2: FinSecure Financial Services
FinSecure, dealing with sensitive financial data, required a robust spam filtering mechanism to comply with regulatory standards. After integrating a machine learning-based spam filtering system, they not only improved their detection rates but also enhanced customer trust, resulting in a 20% increase in client retention within the first year.
Challenges and Considerations
Despite the numerous advantages of spam filtering machine learning, businesses must navigate several challenges:
1. Initial Setup Costs
The implementation of machine learning systems may involve notable initial investments in technology and human resources. These costs must be weighed against long-term benefits.
2. Data Privacy Issues
Organizations must ensure that any data collected for training machine learning models complies with data protection regulations, such as GDPR. Balancing spam filtering needs with privacy is crucial.
3. Complexity of Implementation
Integrating sophisticated machine learning systems may be challenging for businesses lacking technical expertise. Collaborating with experienced IT professionals or outsourcing to specialized firms may alleviate these concerns.
The Future of Spam Filtering with Machine Learning
Looking ahead, the landscape of spam filtering will continue to evolve with advancements in machine learning technologies. Future trends may include:
- AI-Driven Solutions: The integration of artificial intelligence will enhance the decision-making capabilities of spam filters.
- Natural Language Processing: Improved language understanding will lead to better contextual spam detection, minimizing false positives.
- User-Centric Filtering: Systems that adapt to individual user preferences will enhance overall email management.
Conclusion
In conclusion, the adoption of spam filtering machine learning represents a significant advancement in the quest for effective email security. With its ability to learn, adapt, and significantly reduce spam, organizations can enhance their operational efficiency and safeguard against online threats. As we continue to embrace technological innovations, investing in machine learning solutions for spam filtering will undoubtedly pay dividends, leading to a more secure and productive business environment.
For businesses seeking to enhance their IT services and security systems, explore how Spambrella can provide tailor-made machine learning solutions to combat spam effectively.